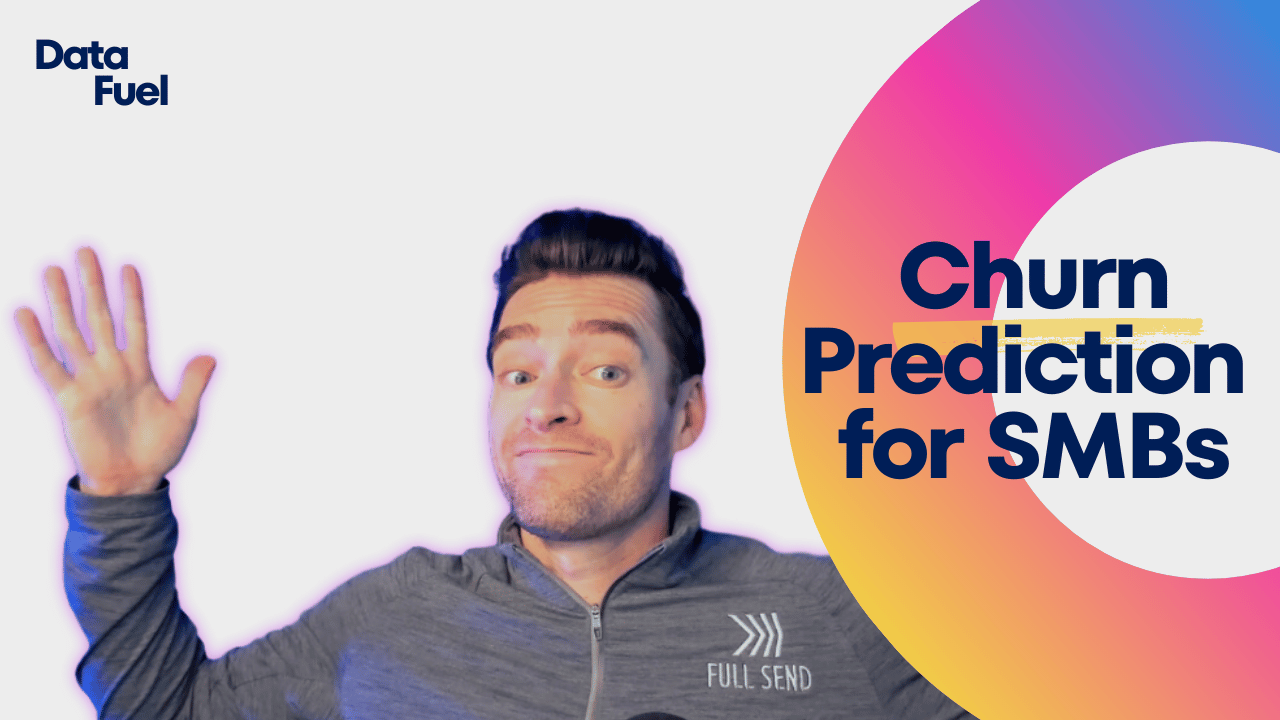
Introduction
What if you could predict when customers are about to leave before they even make the decision themselves?
"Customer churn prediction is actually one of the most accessible ways for SMBs to get started in data science."
Today, we’re exploring how small and medium-sized businesses (SMBs) can leverage data science to predict customer churn, retain customers, and boost revenue.
Why Churn Prediction is a Strong First Step in Data Science
Churn prediction is a great starting point for SMBs because it helps businesses proactively address retention issues.
"How do you help your team proactively address retention issues? How do you give visibility into which customers need extra attention?"
By identifying customers at risk of leaving, companies can tailor engagement strategies and optimize their customer experience.
Industry-Specific Churn Indicators
Churn indicators vary by industry, and identifying these key signals can help businesses develop effective retention strategies.
SaaS
- Declining feature usage
- Fewer logins
- Increased support tickets
E-commerce
- Decrease in order frequency
- Abandoned carts
- No email engagement
Subscription Services
- Payment failures
- Skipped renewals
- Reduced engagement
Professional Services
- Fewer inbound inquiries
- Reduced email response rates
- Lower invoice volume
"If customers start skipping shipments or not engaging in your email cadences, that’s a sign they may churn."
Capturing the Right Data for Churn Prediction
Before implementing advanced churn prediction models, businesses must start with simple metrics:
- Last purchase date
- Support interactions
- Product usage trends
Establishing a strong data foundation is crucial for actionable insights.
Strategies to Reduce Churn
Re-engagement Campaigns
- Implement onboarding campaigns to boost early engagement.
- Personalize outreach for customers who show early signs of disengagement.
Product Experience Enhancements
- Improve feature accessibility.
- Introduce guided tutorials or automated reminders.
"Let’s implement a better onboarding campaign. Let’s increase engagement in the initial experience with our product."
Building a Churn Prediction Model
Step 1: Identify a Control Population
Analyze historical data of churned vs. retained customers to find key trends.
Step 2: Identify Churn Levers
Determine which behaviors signal a high risk of churn, such as login frequency or support interactions.
Step 3: Train a Machine Learning Model
Use historical churn data to create a predictive model that identifies at-risk customers.
Step 4: Validate the Model
Test the model’s accuracy by comparing predictions against actual churn rates.
Step 5: Implement and Automate Actions
Integrate the model into your CRM and automate actions such as targeted email campaigns or support outreach.
Example: Churn Prediction in Gym Memberships
Gyms track member check-in data to predict churn.
"If a gym notices two months of non-attendance, they might send personalized outreach to re-engage members before they cancel."
Applying similar strategies across different industries can yield higher retention rates and revenue growth.
Tools to Get Started with Churn Prediction
- Stripe Sigma: SQL-based tool for analyzing transactional data
- CRM Integrations: Automate retention strategies based on churn risk
- Custom Data Models: Work with a data analyst to develop a predictive model tailored to your business
Common Pitfalls in Churn Prediction
1. Relying on Too Few Data Points
A narrow dataset limits the ability to identify meaningful patterns.
2. Failing to Act on Insights
Simply identifying churn risk isn’t enough—businesses must take action to prevent it.
3. Treating All Customers the Same
Personalized engagement is key. Tailor retention strategies based on customer segments.
Final Thoughts
Churn prediction is a powerful and accessible way for SMBs to harness data science. By tracking key indicators, analyzing patterns, and implementing automated retention strategies, businesses can reduce churn, increase customer loyalty, and drive profitability.
"If you can start using features like tagging and automation to capture data and trigger actions, you’re taking the first step toward turning insights into value."
Take the time to analyze your customer churn patterns and explore ways to apply these insights. The result? Stronger customer relationships and a more profitable business.